What ChatGPT is bad at…and what to use instead
ChatGPT is getting a lot of attention lately, but it’s not the solution for everything. Why? It’s a system that makes up facts.
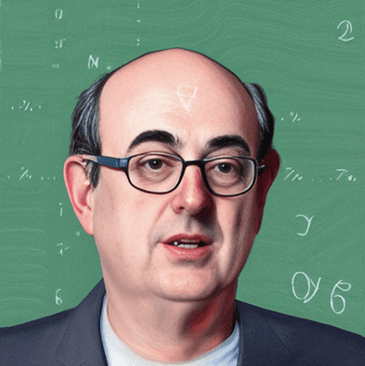
“Stephen Wolfram thinking in formal languages” – Artwork created by Generative AI (Stable Diffusion)
Consider the following query:
What is the price of a movie ticket in Silver Spring vs. Seattle?
ChatGPT is a terrible choice for finding an answer to this question. But why exactly is that?
The prime reason is that “[ChatGPT] may make up facts.” This is according to Mira Murati, CTO of the system’s creator, OpenAI.
ChatGPT was trained with humongous amounts of text. It searches for textural content relating to the question and recombines it. This leads to good little “essays” about a topic, a distillation of what could be called the “consensus of the world’s knowledge.” This has excellent practical use cases; it represents the next level of world knowledge research after search engines. It is often correct because, in an almost “democratic” way, the majority opinion will dominate outliers.
Why is it so bad, then, for a seemingly simple question such as the above?
ChatGPT’s limitations
First, it generally doesn’t give us the age of the data it uses for its answers, so we don’t know whether it is current enough for our purpose. The current version of ChatGPT is trained with data only up to 2021. To the system’s credit, for this particular question, it says so in the answer.
But even if it was trained on newer data (as it should be soon), secondly, it generally doesn’t give the information source so we can assess its trustworthiness. It can’t because it doesn’t work by selecting a single source.
Third, it does not allow verification of names, which can be ambiguous. For the query, “What is the population of Frankfurt?,” it gives the response for the larger Frankfurt am Main, not the smaller Frankfurt an der Oder. And it doesn’t indicate that specification anywhere in the response.
More generally, the fourth point is that ChatGPT does not allow verification of whether the natural-language query was understood correctly. For example, a more complex question such as, “What are the colors that a largemouth bass can see?,” ChatGPT’s response is wrong and can only be described as rambling nonsense. Bass only have two of the three color cones that humans have – red and green, not blue.
Finally, the fifth limitation is we have no way to understand how the output was manipulated by the creators of the system. In the same interview quoted above, Murati points out that “there are questions about how you govern the use of this technology globally. But we [OpenAI]…need a ton more input…that goes beyond the technologies – definitely regulators and governments and everyone else.” Making AI use “safe” is the stated goal of OpenAI, which is of course an honorable goal. However, there is no transparency at all about how that works, which is problematic for a system that is consulted by people for unmitigated truth.
Are you engaging the right half of your computer’s brain?
For questions like these, we are neither looking for a nice “essay” nor a “majority opinion.” We do not want to poll the world for Seattle ticket prices. We want to get the response from a single, reliable source.
Chat GPT does not work that way. It is awful when it comes to hard facts. Its approach can be characterized as “associative,” “creative,” “imaginative,” “brainstorming,” “expressing things verbally,” “finding generally accepted opinion,” “putting small pieces of information together and see how they combine,” “coding following examples and best practices,” “asserting things with no need or no ability for proof,” and so on.
A different kind of thinking is much more appropriate for the questions above. The epitome of that thinking is, of course, doing math. Characteristics include “result correctness is paramount,” “things are either totally correct or totally false,” “this is not a matter of majority opinion,” “strictly logical approach,” “formal language eliminating the uncertainties of natural language,” etc.
Unconsciously, we humans switch our way of thinking based on the task at hand. We put on our “creative thinking cap” or the “formal thinking cap.” In fact, most believe that people are specifically talented in one or the other: She excelled in languages while he was the mathematical type of guy. Many call it right brain/left brain thinking, although science dismissed these categories for being overly simplistic. Others may call it fast thinking/slow thinking, after Kahneman’s book. Others again may call it female/male thinking, though that is a gender stereotype that is certainly outdated.
Of course, psychologists researched this and distinguished many more types of thinking. But it may suffice to distinguish two types in this context. Let’s call them “creative thinking” and “formal thinking.” Asking ChatGPT about ticket prices is using a “creative thinking system” for a question that a “formal thinking system” is much better suited for.
The software equipment to a math professor
Does a “formal thinking system” even exist? Indeed, it does, and probably the best example is Wolfram|Alpha, created by Stephen Wolfram. It accepts natural language queries, like ChatGPT. But it fundamentally works differently by translating the query into a formal representation and then builds the answer from that on a curated set of trustworthy data sources.
It fares much better than ChatGPT for the questions above. It gives a perfect answer to the movie ticket price question, including a description of how the question was interpreted, the data source consulted, and its age.
Asked about Frankfurt, it shows which Frankfurt it’s referring to and even gives the option to select the other city.
It does not know which colors a largemouth bass can see, but importantly, it clearly says so. Finally, its content and response are not influenced by the program’s authors.
Wolfram|Alpha has been around for 10+ years, and the miniscule amount of attention it gets in comparison to ChatGPT seems a bit unfair. However, you may have used it unconsciously because, at one time, it powered search engines Bing and DuckDuckGo as well as conversational AI systems Siri and Alexa, though it doesn’t any longer. Today, when the results from a sample of search engines and conversational AIs are compared, none returns a quality answer that is close to Wolfram|Alpha’s.
Wolfram|Alpha’s limited public attention and application are complicated and have little to do with its capabilities.
This is not an advertisement for Wolfram|Alpha but a simple analogy; as different kinds of human thinking exist, so do different answering systems. Beyond Generative AI, there remains a need for systems that work based on formal descriptions and curated datasets. Humans unconsciously change their thinking hats. Using AI, we should learn to use the right system based on the nature of the query we have. This is part of AI literacy that we all urgently need to develop in order to thrive in the Generative AI future.
The billion-dollar question: Is ChatGPT overrated?
In light of all this, will the current hype around ChatGPT wane? Should we expect another “AI winter,” as we call similar events in the past?
No, we should not. Although ChatGPT (and Generative AI in general) is not the right tool for all kinds of questions, it still represents a massive advance for a huge class of questions, especially creative thinking tasks we thought to be at the epitome of human thought. In an interview, Sam Altman, CEO of OpenAI comments on the kind of thinking AI excels at:
“I think we’re seeing this now as tools for creatives, that is going to be the great application of AI in the short term…I think it’s interesting that if you ask people 10 years ago about how AI was going to have an impact, with a lot of confidence from most people, you would’ve heard, first, it’s going to come for the blue-collar jobs working in the factories, truck drivers, whatever. Then it will come for the low skill white collar jobs. Then the very high skill, really high IQ white collar jobs, like a programmer or whatever. And then, very last of all and maybe never, it’s going to take the creative jobs. And it’s going exactly the other direction. There’s an interesting reminder in here generally about how hard predictions are, but more specifically about we’re not always very aware, maybe even ourselves, of what skills are hard and easy.”