In Data We Trust: Smart Data Management in Enterprise Architecture
Discover how smart data-driven governance transforms Enterprise Architecture, empowering organizations to be more efficient, reduce risks, and increase profits.
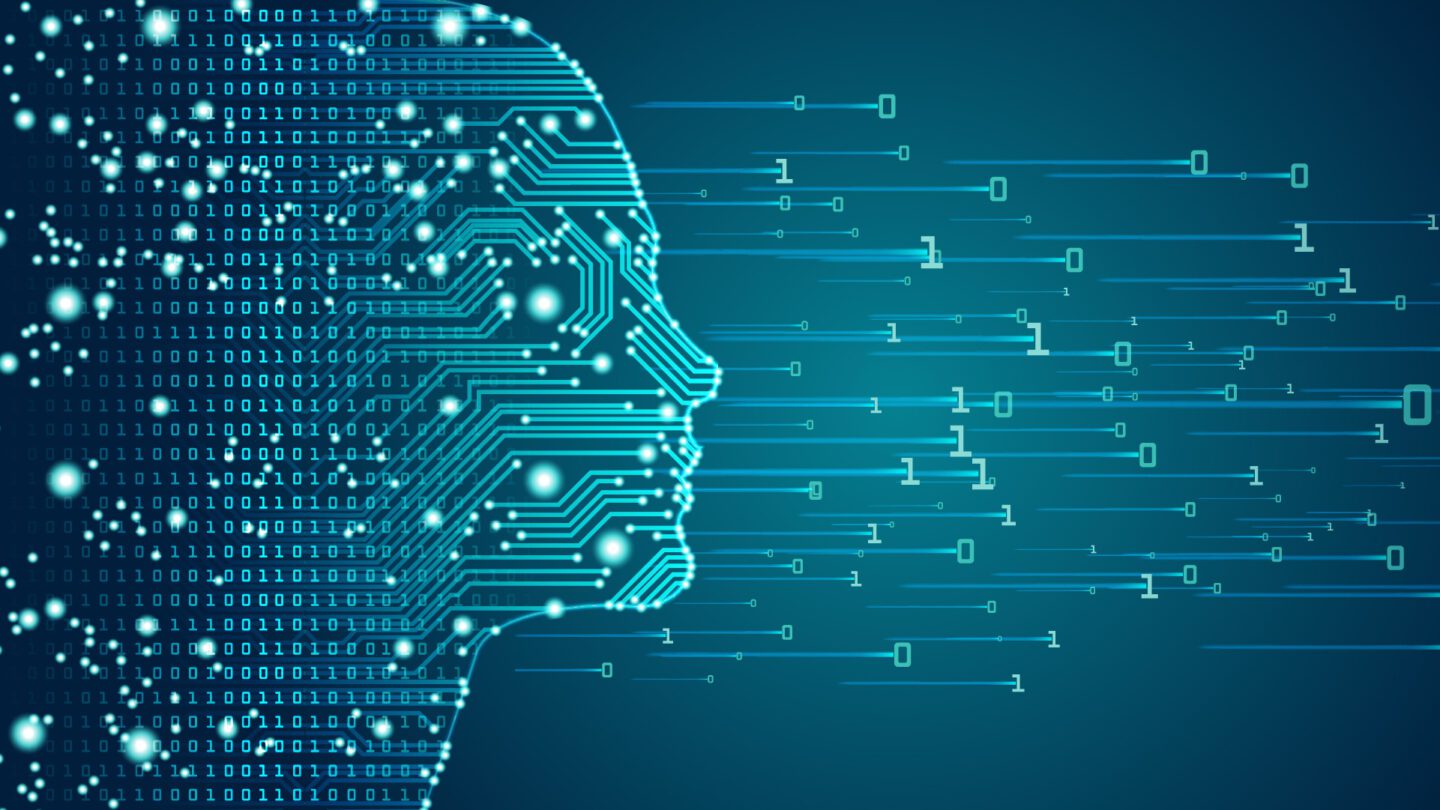
In today’s fast-paced business landscape, data reigns supreme. Good data is an invaluable asset for driving organizations to succeed. Leveraging data and the insights it provides is not just a choice but a necessity for informed business planning and decision-making.
US statistician W. Edwards Deming once said, “In God we trust. All others must bring data.” But trusting in bad data fooled humankind more than once. Cristopher Columbus’s navigation was a bit off-track due to inferior routing calculation. The Mars Climate Orbiter NASA space probe got “lost in space” — a $193 million dollar mistake because it used two different measurement systems. And overstated values in real estate data lead to a world financial meltdown in 2008.
Incomplete, siloed, duplicated, inconsistent, outdated, and inaccurate data is the arch-nemesis of progress. Low-quality data leads to incorrect conclusions and misinformed decisions. Duplications and redundancies waste time and resources, while flawed projections result in missed opportunities and revenue loss. Inaccurate reporting often leads to compliance nightmares. It’s clear that the value of good data cannot be overstated.
Most of us will never discover a new country or planet but it is interesting to reflect on how the data we use every day could ever become so randomly consequential. We can certainly try our best to make sure that the data we handle and produce is high quality.
Is your Enterprise Architecture smart data-driven?
It is the responsibility of Enterprise Architecture (EA) to provide a 360-degree view of your organization, support data-informed decision-making, and, ultimately, facilitate better business outcomes. But how can we ensure that the data we base our decisions on is reliable enough to pave the way from data-informed to data-driven EA?
Data integration and automation are key to establishing a single source of truth. By ensuring that data is connected across sources, you eliminate information silos and identify data gaps that can hinder effective decision-making.
The alignment of IT and business strategies is essential to meet data needs and changes in the present and in the future. Mapping EA data against business strategies and initiatives ensures alignment and visibility into data dependencies and helps you to understand how your business goals are connected to your applications and technology infrastructure. This can provide you with the insights needed to align these components effectively and perform impact and scenario analysis across business and IT domains, inevitably facilitating effective transformation and IT investment portfolio management.
Data management is a collaborative effort. Decision-makers and business stakeholders are directly impacted by data and data quality. Stakeholder collaboration is needed to meet expectations, ensure cross functional integration and system functionality, and define data needs and accessibility. Additionally, it is imperative to provide business-relevant analytics to drive organizations toward informed business decisions and investments.
Data analysis and data management is a dynamic process, so what is needed to master it?
5 essential tasks for smart data-driven governance and decision making
- Collect data — Collaboratively set goals for the insights required, KPIs, and the associated data to measure the effectiveness of your data-driven approach. Establish a single source of truth in a central repository, eliminate data silos, and ensure a continuous flow of data.
- Simplify data management — Deploy ‘smart data workbenches’ and provide at-a-glance object information as well visualization for easy, efficient, and fast data management.
- Ensure data quality management — Identify bad data before it enters the system and capture ongoing quality issues with data quality rules. Take the data dimensions, indicators, and thresholds into consideration. Improve data quality over time and ensure compliance with data standards and policies.
- Enable collaborative data management — Ensure stakeholder involvement and accountability with fostering data collaboration, establishing clear responsibilities as well as allocating tasks and requests.
- Continuously analyze and optimize data — Perform rapid portfolio, lifecycle, and data quality analysis to keep data current, eliminating redundancies and inconsistencies. Leverage visualizations for effective communication, presentation, reporting, and constant reassessment to improve and maintain the value of your data.
EA provides the essential foundation for data-informed, optimized decision-making across organizations. Smart EA data management brings it to the next level — data-driven — to transform data into strategic insights, realizing the promise of data as a key business differentiator. Easy access to the right data at the right time improves efficiency, lowers costs, and reduces risks. It also helps to predict internal and external changes and path the way for more innovation and increased profits.
Find out more about how to pave the way to data-driven Enterprise Architecture and watch our Alfabet EA tool in action during our webinar: Leverage smart data management to empower your Enterprise Architecture.