Integrating AI within your Enterprise
There simply is no “one-size-fits-all” approach to integration.
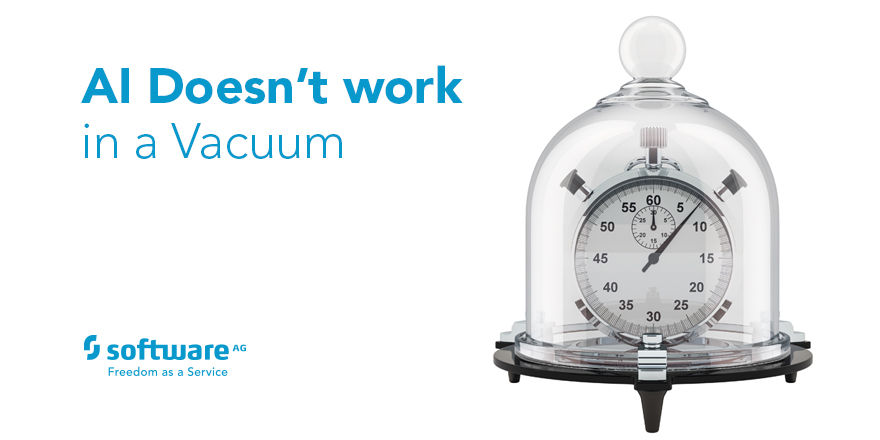
Think back to school and science class: You probably conducted an experiment where you placed an alarm clock (set to go off in 5 minutes) under a glass jar and the teacher pumped all the air out of the jar. When the alarm went off, you couldn’t hear it, right? Without air, sound does not travel – nature abhors a vacuum.
This is also true of artificial intelligence – it cannot survive in a vacuum and needs a rich ecosystem of data where it can thrive. This can only be achieved by integrating “trustworthy AI” systems with the rest of an organization’s IT landscape.
I had the pleasure of delivering a keynote at the European Big Data Value Forum 2019 in Helsinki recently and couldn’t help noticing that many other contributions were focused on only two aspects of AI or Big Data: They either referenced highly specific and isolated use cases or they talked about somewhat lofty initiatives at a very high level, for instance, pan-European or smart city data spaces.
Literally no one was speaking about the (mundane but unavoidable) task of connecting all these data sources. So, I decided to give the audience some concrete architectural guidance drawn from our decades of experience.
I segmented the vast realm of integration requirements into three dimensions calling for three different architectural approaches:
- Hybrid Integration
Connecting classical on-premises IT systems to a tsunami of micro-applications all cloud-based and delivered as SaaS, requires more than just a single integration component (e.g., an Enterprise Service Bus) in the cloud or on premises. Due to different non-functional requirements this needs a distributed integration Platform as a Service (diPaaS) where organizations can pick and choose where to deploy their integration artifacts and assets.
- IT/OT convergence
For some, “integrating” the machines and devices of automation or operational technology (AT and OT), or the “Things” of the IoT, simply amounts to linking the end-points to a central cloud-based IoT platform. For the very first steps, this is enough, but most industrial IoT customers, for instance, require a distributed IoT architecture. This includes suitable edge components to stay within their stringent latency, bandwidth, and security requirements.
- Knowledge integration
Historically, tasks like building a complex integration flow were handed down to IT specialists, with well-known delivery difficulties (slow, cumbersome, expensive). Obviously, this approach will no longer scale when you need to connect thousands of individual devices to your IoT platform.
Equally, many tasks need domain experts with deep knowledge in an iterative setting, for instance, developing an alarm for paint robots or identifying anomalies and establishing correlating early-warning indicators. They need to be enabled to do this themselves with self-service analytics, instead of requiring them to learn and program SQL (or some other) code.
No one-size-fits-all
Software AG understands that different enterprises have different requirements in each of the three dimensions. There simply is no “one-size-fits-all” approach to integration. So, we do not force organizations into the cloud, we do not mandate any compulsory on-premises component.
On the contrary, we let your non-functional requirements determine the optimal distributed integration architecture. And this we call “freedom as a service.”