How to successfully deploy machine learning models quickly into the hands of operational experts
As organizations look to do more – or even the same amount – of work with fewer resources, learn how to bridge the gap between data scientists and operational experts.
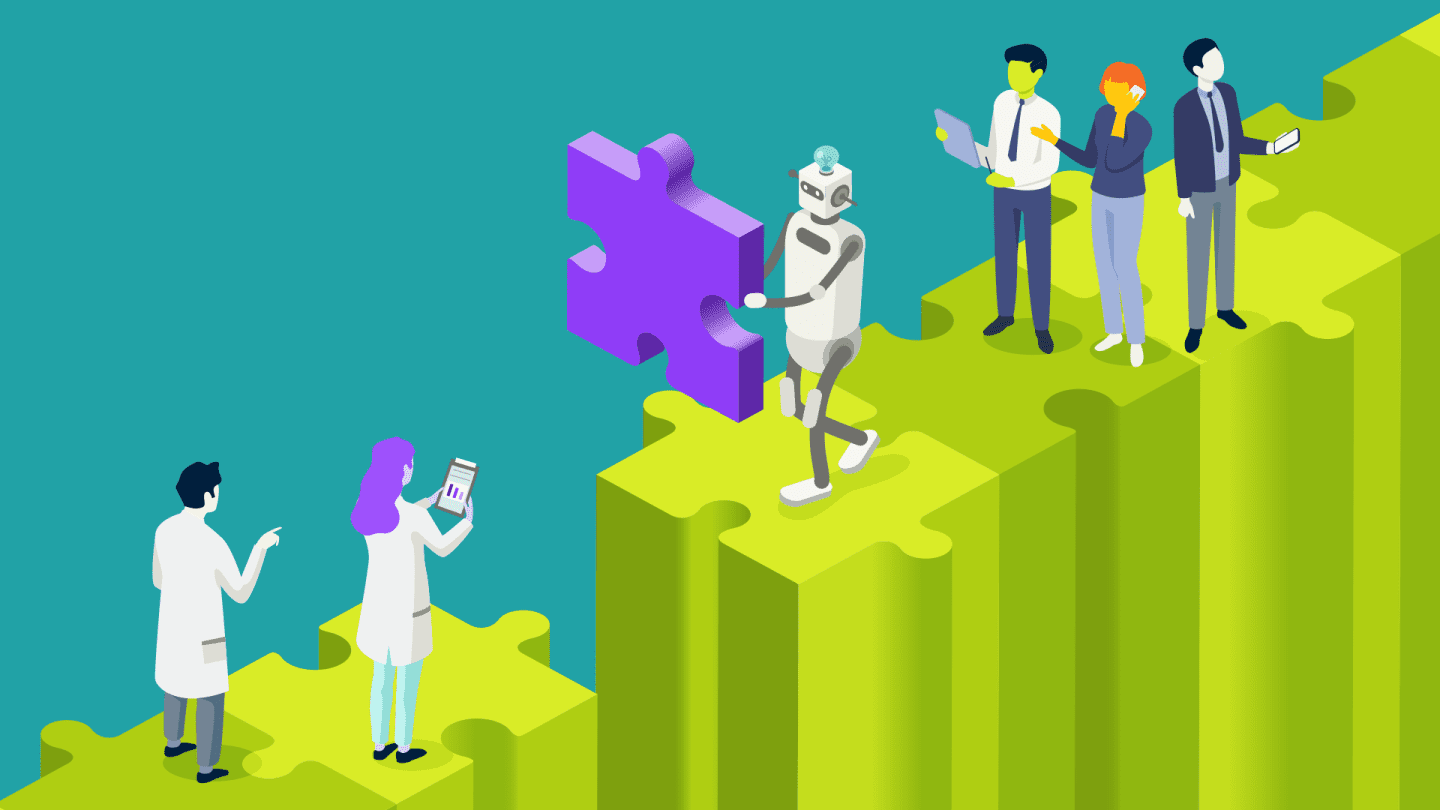
A common pain point we hear about from our customers is that there is a wide gap between what their data scientists can do and what their operational experts need to know. Data scientist groups are typically charged with looking at organizational challenges from a broad perspective and leveraging technologies like Machine Learning or Artificial Intelligence to find solutions. What they lack, however, is the subject matter expertise on operations or the context of specific KPIs across their business.
Similarly, operational experts possess expert knowledge about their manufacturing processes and are very attuned to the things that are going to affect their processes. But they don’t have – nor are they expected to have – the data science expertise to build, train, or deploy machine learning models into operations.
In a perfect world, an organization would pair a data scientist with an operational expert to solve a specific problem. Many times, this is the case. But as organizations look to shore up their resiliency in the face of uncertain times, leaders are asking employees to do more with less, and quickly. So, how can we bridge this gap between data scientists and operational experts?
Power of Machine Learning in Use: An Anomaly Detection Use Case
There may be several reasons operational experts want to collaborate with central data science teams on a data science project. For the operational expert, machine learning can be defined as the application of algorithms and statistical models to analyze and predict important process parameters as a function of one or more independent variables. One such use case is stronger anomaly detection.
Some anomalies are much more difficult to detect and predict. In certain situations, for example, an anomaly might occur even when a process appears to be functioning normally. For these more involved cases, data scientists can apply a machine learning technique known as an anomaly detection model. They can identify rare items, events, or observations that deviate significantly from the rest of the time-series dataset and that are known to be outside normal operating behavior. They can be used to set up monitors and alerts when special deviations occur.
In fact, an anomaly detection model can help prevent a complete plant shutdown. At one chemical plant, fluid from one process periodically would leak into a compressor on another process. When this occurred, the compressor eventually would become damaged. They only way to repair the damaged compressor is to shut down the plant entirely. Engineers already had determined that vibrations were causing the leak. However, they had no way to monitor the problem. Furthermore, once the leak started, operational experts were unable to correct the anomaly before the compressor was damaged.
First, engineers installed vibration sensors near the compressor. They then collected time-series data from those sensors and analyzed it for period of good behavior. Data scientists then loaded the data into a “hub” integrated into the industrial analytics solution to train the machine learning model with different types of vibration patterns. Once complete, engineers created a new machine learning model tag from the trained model. Then they activated a monitor on the new tag, which could detect irregular vibrations. Finally, they used an analytics software solution to monitor and alert capabilities to notify key stakeholders.
The new soft sensors helped avoid a complete plant shutdown. When a leak occurred, process experts got an alert with enough time to intervene before it damaged the compressor. And while the machine learning model tag picked up the right kind of vibrations to indicate a problem, engineers would have been unaware of it any other way. All other process parameters appeared normal.
The Power of Democratizing Machine Learning
So how can companies put the power of machine learning in the hands of all operational experts? And improve collaboration between centralized data scientists and local sites to solve more complex use cases and achieve operational excellence?
We believe the best solution is a blended model that uses a federated central group as a facilitator. Individual business units then take responsibility for making continuous improvements. In this environment, centralized groups can deploy machine learning models inside the advanced analytics solution and make them available to everyone in the organization. Simultaneously, data scientists are kept in the loop to help solve the most complex process anomalies.
A smooth symbiosis between process experts and central data science groups is achievable by democratizing machine learning using the right industrial analytics software.
Resolving Challenges Using TrendMiner
TrendMiner bridges the collaboration gap between operations and central data science groups. The Next Generation Production Client now offers an addition to its standard features called MLHub: A notebook environment for deploying machine learning models that help accelerate business, performance, and sustainability objectives. It puts the power of machine learning in the hands of operational experts.
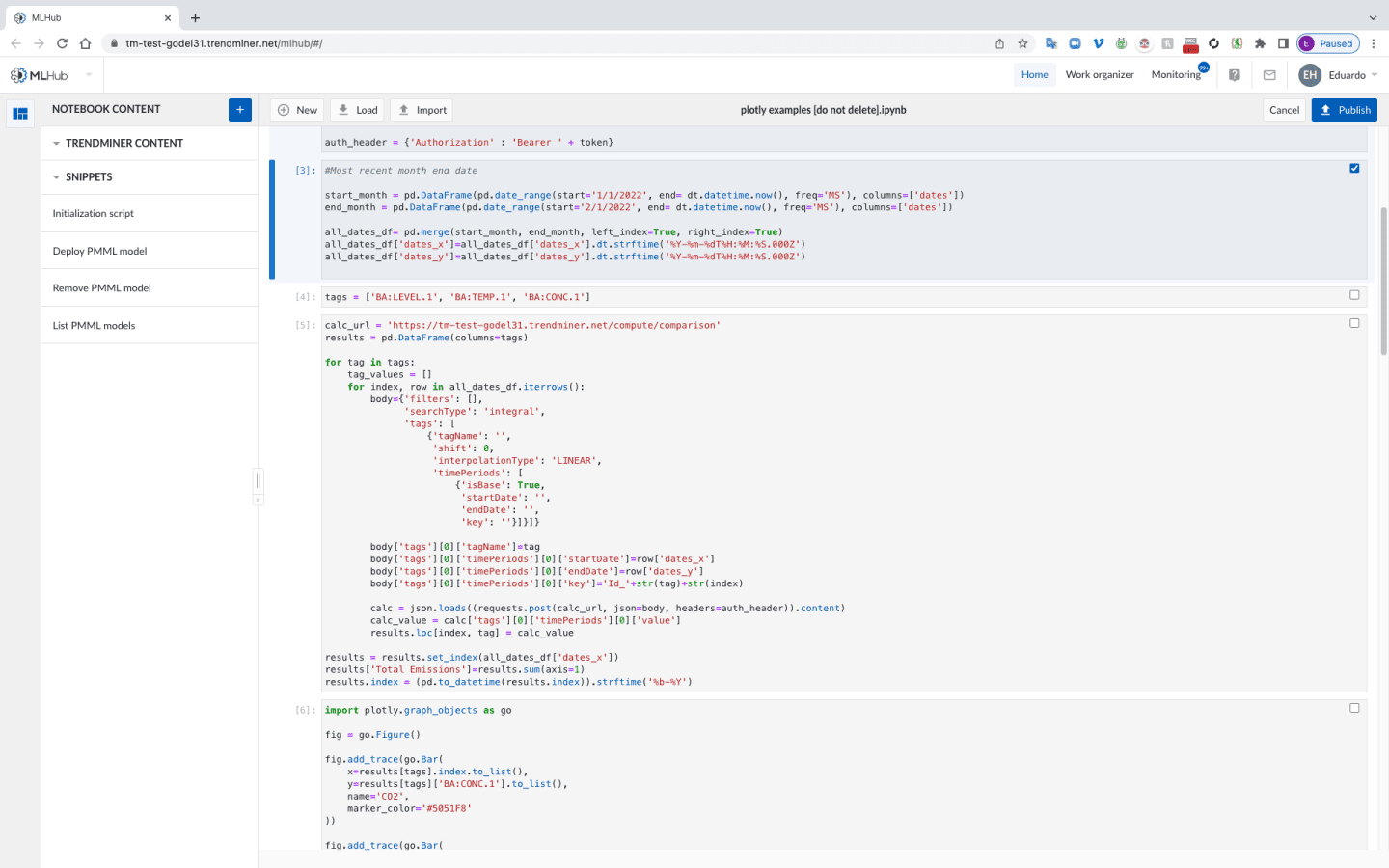
MLHub reduces the demand on central analytics teams. This drastically improves the adoption rate of data science projects as well as reducing the throughput time of those projects. The integrated environment for deploying machine learning models also fosters efficient collaboration between operations and the central team. With data democratized to all software users, they can share and reuse machine learning models and notebooks across the organization.
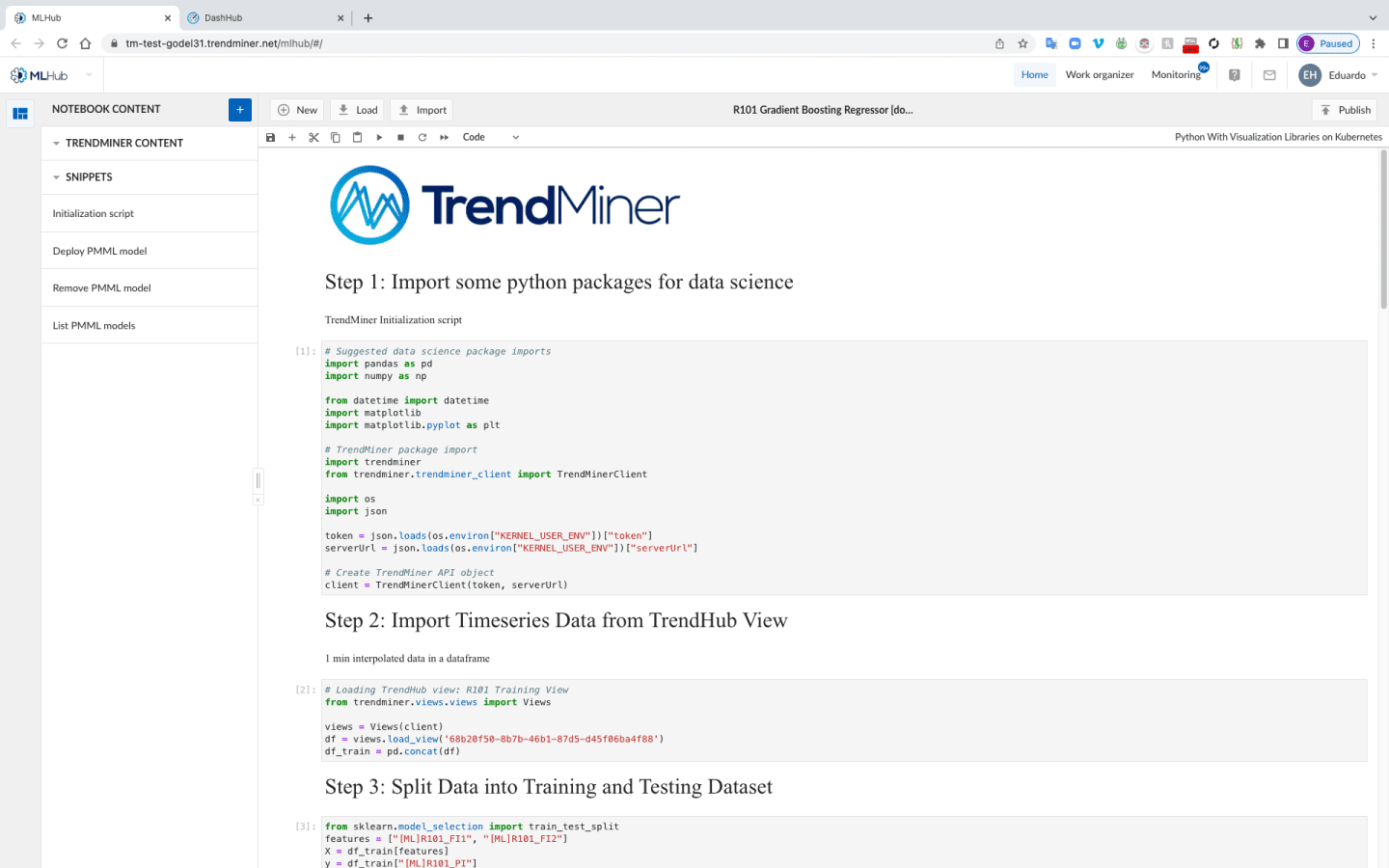
All pre-processed time-series data and its contextual operational information is available to data scientists and operational experts. They can use it to create advanced visualizations and machine learning models, which can be deployed quickly into operations for iterative improvements. Unlike most machine learning platforms or artificial intelligence solutions that keep data locked in silos, TrendMiner empowers engineers to address and solve more complex use cases that provide their company with a competitive advantage.
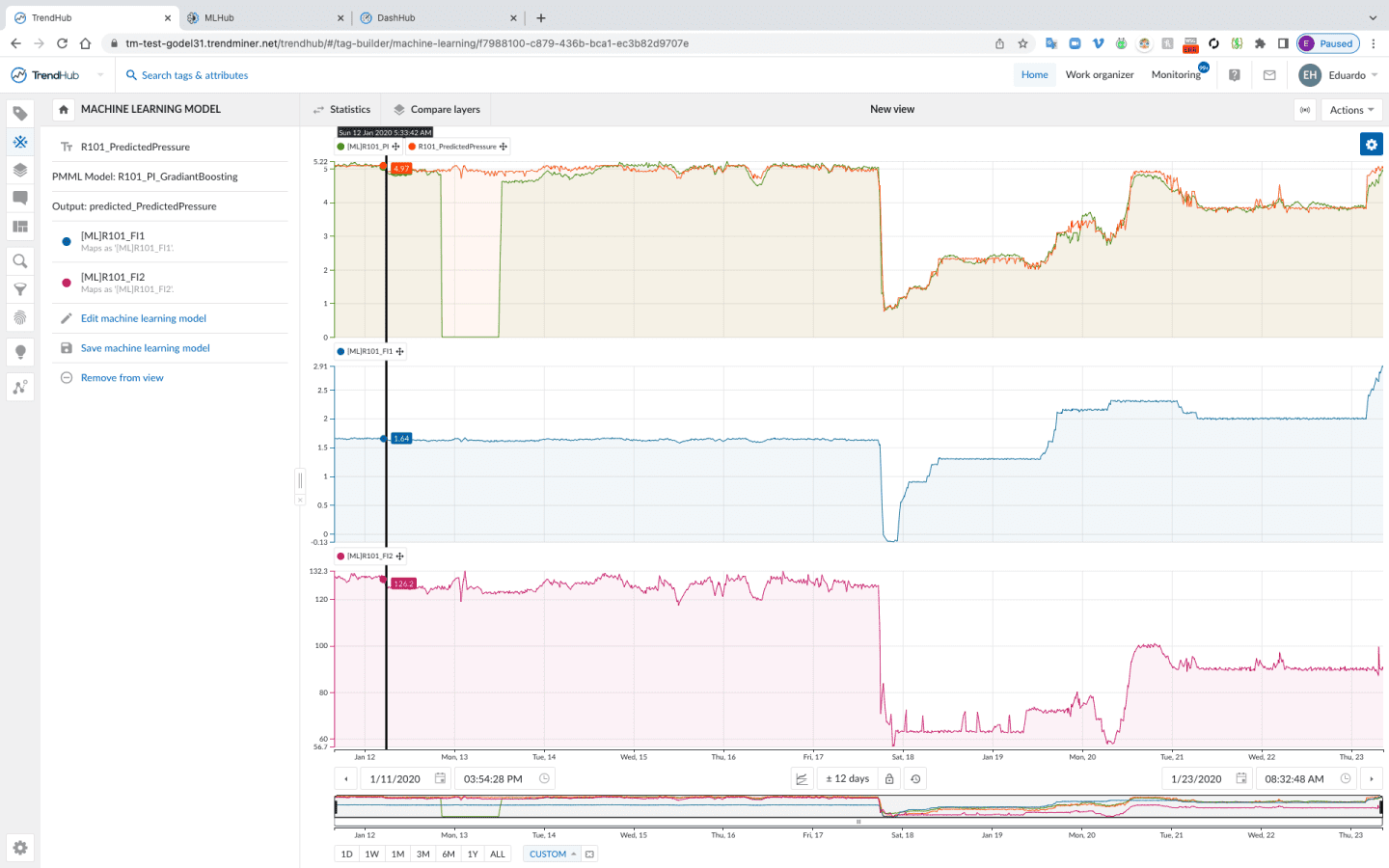
Key Benefits of TrendMiner’s MLHub
MLHub gives data scientists access to open-source libraries that can be used to strengthen anomaly detection. The models and notebook sections can be shared so analysts can train and deploy them quickly.
Operational experts can accelerate a machine learning exercise by preparing time-series data through searching, filtering, and saving DashHub views as input. Data scientists then can use this saved data in TrendMiner to create and train machine learning models in MLHub. Calculations and digital tags can be saved in views for further processing. Machine learning model tags also can be added as new digital tags to apply all TrendMiner capabilities.
MLHub can also work with contextual data. Contextual data can be the saved results of process monitoring or information contained in third-party business applications (such as batch run data, computerized maintenance management systems, and laboratory management systems). These items that help put operational performance into context also can be used as input for machine learning models. Conversely, the output of machine learning models can be saved as contextual data for additional insights.
Data scientists and engineers can see notebook cell outputs as tiles in dashboards. They can also generate interactive graphs that can be shown directly on dashboards and display forecasted model outputs on value tiles (such as for predictive maintenance).
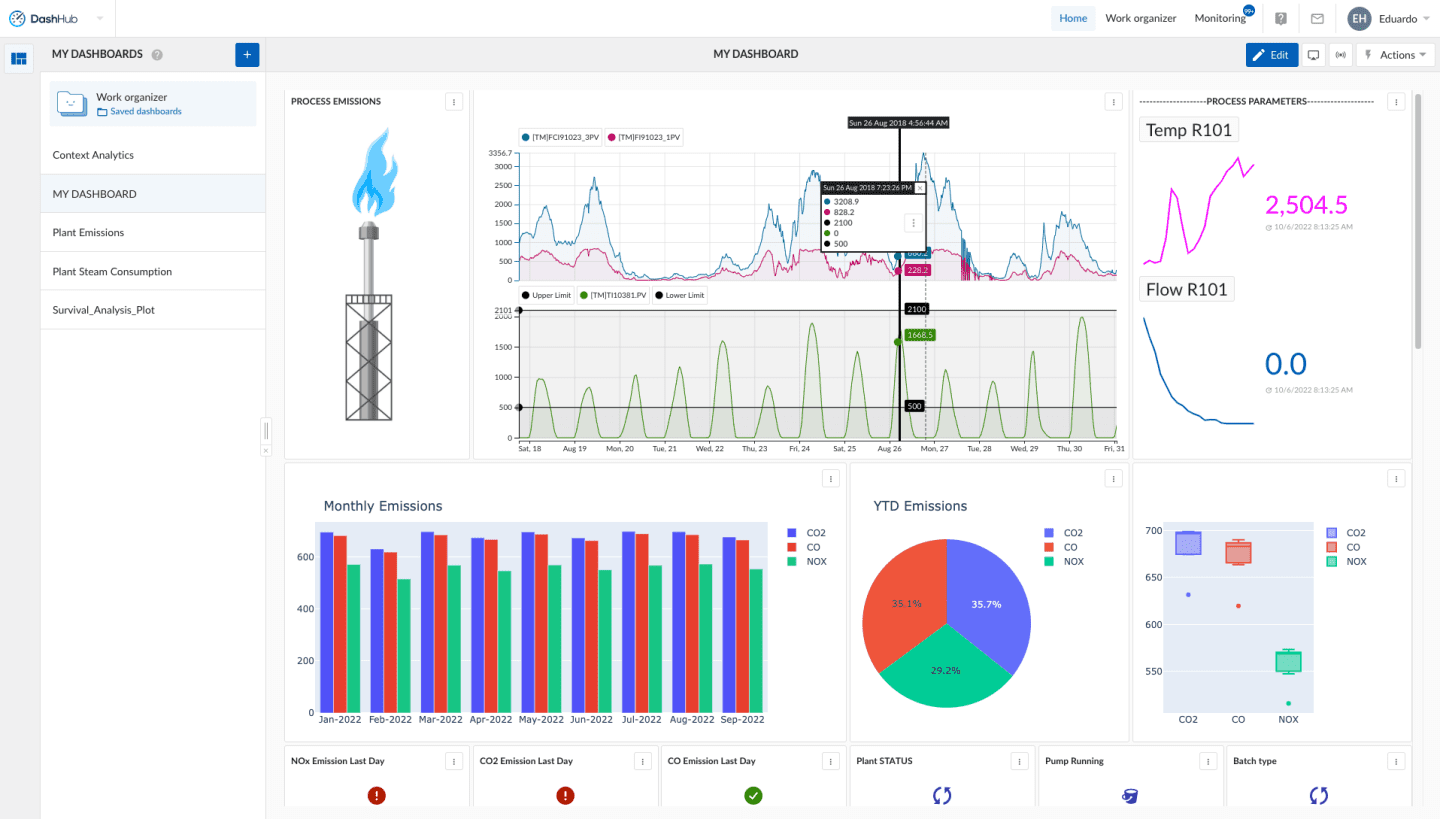
Conclusion
Companies that use TrendMiner can be sure that improvement projects will not be left on the table. Through its MLHub, the software places machine learning in the hands of every process engineer.
The result?
- Better collaboration between engineers and data scientists,
- More efficient use of central groups and operational experts,
- Advanced anomaly detection and monitoring models,
- An improved competitive edge, and
- Increased organizational resiliency and operational excellence.
Deploying machine learning models to analyze, monitor, and predict process behavior has never been easier.