Design your IoT solution to scale for success
Achieve success at scale by implementing data management best practices.
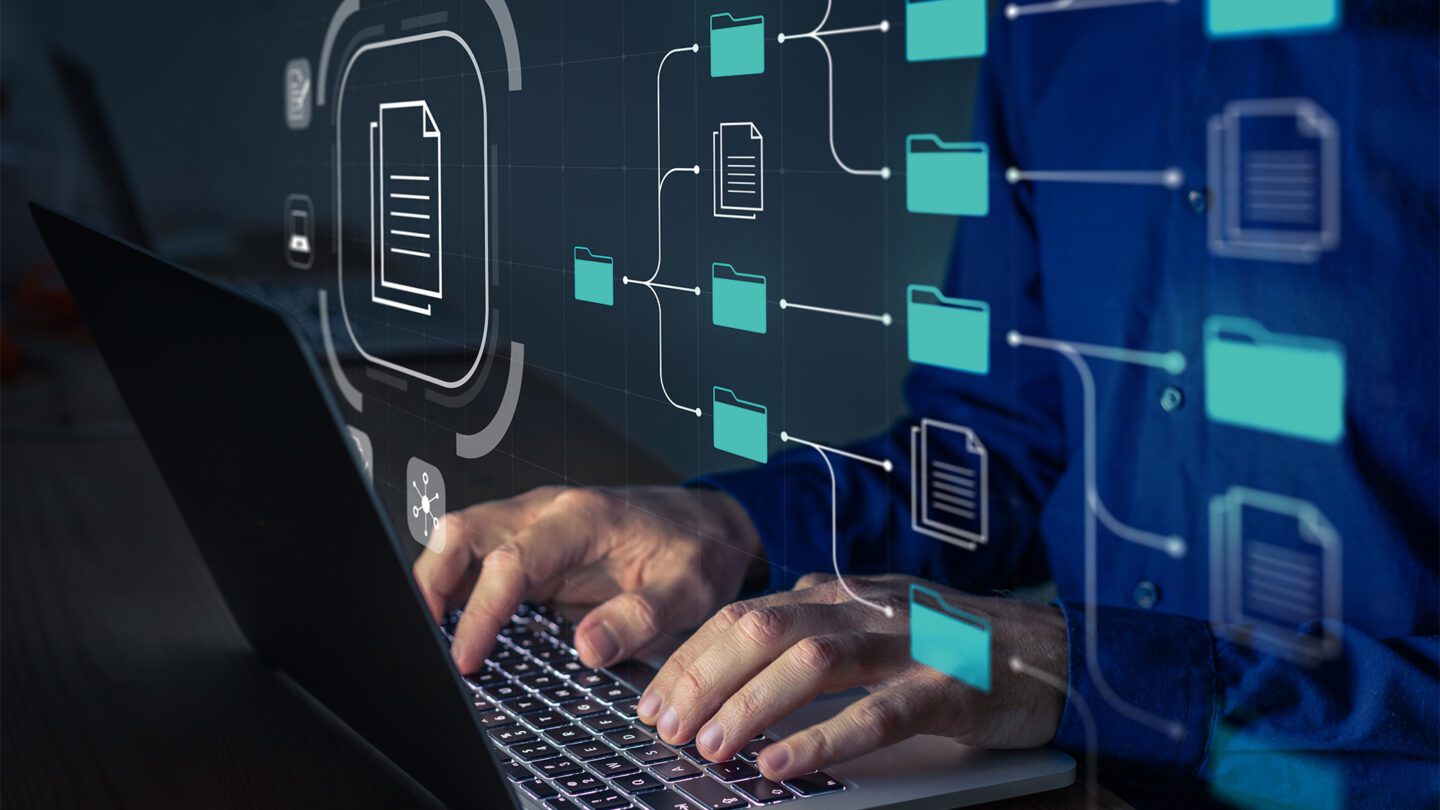
The Internet of Things (IoT) is transforming the way we live, work, and interact with the world around us. For manufacturing and industrial enterprises, IoT has enabled innovative new solutions to improve operations, from introducing smart equipment to condition-based and predictive maintenance to smart logistics. Organizations are now moving beyond experimenting with and piloting solutions and deploying IoT at scale. According to a recent report from Beecham Research, nearly half of organizations have an IoT deployment with more than 500 devices, and more than 50% of enterprises plan to increase the size of their largest deployment by at least 20% over three years.
Data management is critical for scaling IoT solutions
IoT devices generate vast amounts of data, including sensor data, network data, user data, and device metadata. As IoT solutions scale, data management becomes increasingly complex, and organizations must adopt robust strategies to ensure data quality, security, and accessibility. This data is often stored in different formats and on different platforms, including public clouds, private clouds, edge devices, and gateways. As the number of devices and data sources increase, the volume, velocity, and variety of data also increase exponentially, making it challenging to manage, process, and analyze the data effectively. Poor data management practices can lead to data silos, data inconsistencies, security vulnerabilities, and compliance issues, which can impact business performance and reputation.
Effective data management practices help organizations address the challenges of scaling IoT solutions by providing a unified view of data, ensuring data quality, security, and privacy, optimizing data processing, and enabling data-driven decision-making. By adopting a data management strategy, organizations can unlock the full potential of IoT data and gain valuable insights into their operations, customers, and markets.
When considering best practices to effectively manage IoT data, you can consider the key challenges that occur during three phases of IoT deployments:
- Designing solutions
- Managing solutions
- Integrating and using the data gathered
Designing solutions
Centering data along with devices: In the initial phases of designing an IoT solution, device connectivity and device management often take center-stage. This is logical given the value that IoT can provide by improving asset performance. But advancing successfully along the IoT maturity curve requires optimal management not just of the devices but of the data as well. Adopting a data-centric approach ensures that data is collected, stored, and processed in a consistent and standardized format, making it easier to integrate and analyze. The data management strategy should align with business goals, regulatory requirements, and technical capabilities and include data architecture, data governance, data security, data privacy, and data lifecycle management policies.
Implement a scalable data architecture: From the beginning organizations should implement a scalable data architecture that can handle large volumes of data, support multiple data formats, and enable real-time data processing. The initial architecture needs to consider not just the pilot or proof of concept environment. The architecture should also support data integration from multiple sources and enable data analytics and visualization. This will set up the solution for resiliency, stability, and performance.
Managing solutions
Ensure data quality and accuracy: Organizations should ensure that IoT data is accurate, complete, and consistent by implementing data validation, data cleaning, and data enrichment techniques. This ensures that data-driven insights are reliable and actionable.
Ensure data security and privacy: Organizations should implement robust data security and privacy measures to protect IoT data from unauthorized access, theft, or misuse. This includes implementing access controls, encryption, data anonymization, and secure data transfer protocols.
Integrating data
Optimize data processing: Organizations should optimize data processing by implementing data compression, data deduplication, and data caching techniques. This reduces the amount of data transferred over the network, improves data processing speed, and reduces storage costs.
Leverage machine learning and AI: Organizations should leverage machine learning and AI techniques to analyze IoT data and gain valuable insights. This includes implementing predictive analytics, anomaly detection, and recommendation engines to automate decision-making and improve operational efficiency. SMC developed a predictive maintenance solution for pneumatic pumps allowing them to reduce costs from air leakage by 20% and operate equipment more efficiently.
Conclusion
Effective data management is critical for organizations to scale their IoT solutions successfully. By adopting a data-centric approach, implementing a scalable data architecture, optimizing data processing and leveraging machine learning and AI, organizations can unlock the full potential of IoT data and gain valuable insights into their operations, customers, and markets. As IoT adoption continues to grow, so will effective data management practices.